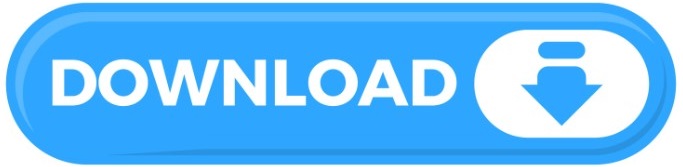

To demonstrate the effectiveness of the proposed method, KITTI datasets is used for comprehensive experiments, and the performance of our approach is tested under different road conditions. The proposed method consists of three main stages: 1) a multi-feature based method is applied to extract feature points 2) a road-segmentation-line-based method is proposed for classifying left and right feature points 3) an iterative Gaussian Process Regression (GPR) is employed for filtering out false points and extracting boundary points.

To solve these problems, a speed and accuracy tradeoff method for LiDAR-based road boundary detection in structured environments is proposed. However, an effective and fast solution for these problems has remained elusive. For road boundary detection in structural environments, obstacle occlusions and large road curvature are two significant challenges. Road boundary detection is essential for autonomous vehicle localization and decision-making, especially under GPS signal loss and lane discontinuities.
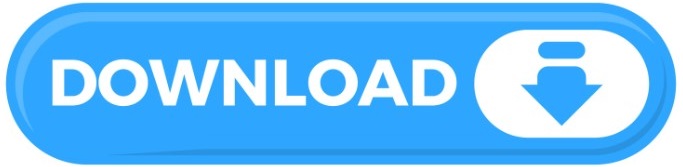